{
"cells": [
{
"cell_type": "code",
"execution_count": 2,
"id": "282817dd",
"metadata": {
"ExecuteTime": {
"end_time": "2023-06-27T20:08:23.900532Z",
"start_time": "2023-06-27T20:08:22.963157Z"
},
"slideshow": {
"slide_type": "skip"
}
},
"outputs": [],
"source": [
"import numpy as np\n",
"\n",
"def print_info(a):\n",
" \"\"\" Print the content of an array, and its metadata. \"\"\"\n",
" \n",
" txt = f\"\"\"\n",
"dtype\\t{a.dtype}\n",
"ndim\\t{a.ndim}\n",
"shape\\t{a.shape}\n",
"strides\\t{a.strides}\n",
" \"\"\"\n",
"\n",
" print(a)\n",
" print(txt)"
]
},
{
"cell_type": "code",
"execution_count": 3,
"id": "3fbf8e4a",
"metadata": {
"ExecuteTime": {
"end_time": "2023-06-27T20:08:26.353134Z",
"start_time": "2023-06-27T20:08:26.089698Z"
},
"slideshow": {
"slide_type": "slide"
}
},
"outputs": [
{
"data": {
"text/plain": [
"array([[2, 4, 6],\n",
" [2, 4, 6]])"
]
},
"execution_count": 3,
"metadata": {},
"output_type": "execute_result"
}
],
"source": [
"a = np.array([1, 2, 3])\n",
"b = np.array([[1, 2, 3], [1, 2, 3]])\n",
"a + b"
]
},
{
"cell_type": "markdown",
"id": "7f116ff0",
"metadata": {
"slideshow": {
"slide_type": "fragment"
}
},
"source": [
"## Broadcasting\n",
">The term broadcasting describes how NumPy treats arrays with different shapes during arithmetic operations"
]
},
{
"cell_type": "markdown",
"id": "f3d30c66",
"metadata": {
"slideshow": {
"slide_type": "slide"
}
},
"source": [
"## Array operations"
]
},
{
"cell_type": "markdown",
"id": "be283ee9",
"metadata": {
"slideshow": {
"slide_type": "fragment"
}
},
"source": [
"> NumPy operations are usually done on pairs of arrays on an element-by-element basis. Arrays of the same size are added element by element"
]
},
{
"cell_type": "code",
"execution_count": 4,
"id": "f272c2e6",
"metadata": {
"ExecuteTime": {
"end_time": "2023-06-27T20:08:36.530322Z",
"start_time": "2023-06-27T20:08:36.521998Z"
},
"slideshow": {
"slide_type": "fragment"
}
},
"outputs": [
{
"name": "stdout",
"output_type": "stream",
"text": [
"[0 2 4 6]\n",
"[1 3 5 7]\n"
]
}
],
"source": [
"x = np.array([0, 2, 4, 6])\n",
"y = np.array([1, 3, 5, 7])\n",
"print(x)\n",
"print(y)"
]
},
{
"cell_type": "code",
"execution_count": 5,
"id": "96b821a8",
"metadata": {
"ExecuteTime": {
"end_time": "2023-06-27T20:08:37.005874Z",
"start_time": "2023-06-27T20:08:36.985938Z"
},
"slideshow": {
"slide_type": "fragment"
}
},
"outputs": [
{
"data": {
"text/plain": [
"array([ 1, 5, 9, 13])"
]
},
"execution_count": 5,
"metadata": {},
"output_type": "execute_result"
}
],
"source": [
"x + y"
]
},
{
"cell_type": "markdown",
"id": "d1c4aa4a",
"metadata": {
"slideshow": {
"slide_type": "slide"
}
},
"source": [
"in numpy, we can just as easily add a scalar to x"
]
},
{
"cell_type": "code",
"execution_count": 6,
"id": "5a475ee0",
"metadata": {
"ExecuteTime": {
"end_time": "2023-06-27T20:08:39.241200Z",
"start_time": "2023-06-27T20:08:39.233969Z"
},
"slideshow": {
"slide_type": "fragment"
}
},
"outputs": [
{
"name": "stdout",
"output_type": "stream",
"text": [
"[0 2 4 6]\n"
]
}
],
"source": [
"print(x)"
]
},
{
"cell_type": "code",
"execution_count": 8,
"id": "c609b563",
"metadata": {
"ExecuteTime": {
"end_time": "2023-06-27T20:08:39.802413Z",
"start_time": "2023-06-27T20:08:39.793716Z"
},
"slideshow": {
"slide_type": "fragment"
}
},
"outputs": [
{
"data": {
"text/plain": [
"array([3, 5, 7, 9])"
]
},
"execution_count": 8,
"metadata": {},
"output_type": "execute_result"
}
],
"source": [
"x + 3 # why added to the full array and not just to the first element? --> broadcasting"
]
},
{
"cell_type": "code",
"execution_count": 9,
"id": "782d1a33",
"metadata": {
"ExecuteTime": {
"end_time": "2023-06-27T20:08:42.052098Z",
"start_time": "2023-06-27T20:08:42.042180Z"
},
"slideshow": {
"slide_type": "fragment"
}
},
"outputs": [
{
"name": "stdout",
"output_type": "stream",
"text": [
"Inefficient [3 5 7 9]\n",
"\n",
"Inefficient [3 5 7 9]\n"
]
}
],
"source": [
"# Alterenative \n",
"# that no one does, hopefully\n",
"# Make a new copy of array\n",
"# Loop through array and add 3\n",
"newx = x.copy()\n",
"for i in np.arange(x.size):\n",
" newx[i] = newx[i] + 3\n",
"print('Inefficient', newx)\n",
"\n",
"print()\n",
"\n",
"# Stretch out 3 to the same shape of array\n",
"# Add x + 3\n",
"new3 = [3, 3, 3, 3]\n",
"x = x + new3\n",
"print('Inefficient', x)"
]
},
{
"cell_type": "markdown",
"id": "85c8fa78",
"metadata": {
"ExecuteTime": {
"end_time": "2023-06-26T16:39:13.224713Z",
"start_time": "2023-06-26T16:39:13.213500Z"
},
"slideshow": {
"slide_type": "slide"
}
},
"source": [
"## Broadcasting\n",
"\n",
"We can think of broadcasting as an operation that stretches or duplicates the value 3 into the array [3, 3, 3, 3], and adds the results. \n",
"\n",
"The code in the first example is more efficient than that in the first because broadcasting moves less memory around during the addition (3 is a scalar rather than an array)"
]
},
{
"cell_type": "markdown",
"id": "ea75d6f8",
"metadata": {
"slideshow": {
"slide_type": "fragment"
}
},
"source": [
"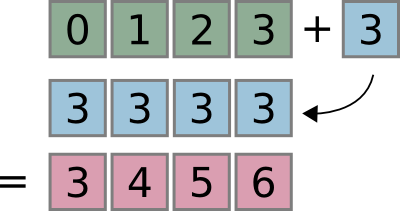"
]
},
{
"cell_type": "markdown",
"id": "8cef6a65",
"metadata": {
"slideshow": {
"slide_type": "slide"
}
},
"source": [
"## 2D broadcasting"
]
},
{
"cell_type": "code",
"execution_count": 10,
"id": "88403cf2",
"metadata": {
"ExecuteTime": {
"end_time": "2023-06-27T20:08:49.074246Z",
"start_time": "2023-06-27T20:08:49.065491Z"
},
"slideshow": {
"slide_type": "fragment"
}
},
"outputs": [
{
"name": "stdout",
"output_type": "stream",
"text": [
"[1 2 3]\n",
"\n",
"[[4 5 6]\n",
" [7 8 9]]\n"
]
}
],
"source": [
"a = np.array([1, 2, 3]) \n",
"b = np.array([[4, 5, 6], [7, 8, 9]])\n",
"\n",
"print(a)\n",
"print('')\n",
"print(b)"
]
},
{
"cell_type": "markdown",
"id": "2fa45377",
"metadata": {
"slideshow": {
"slide_type": "fragment"
}
},
"source": [
"Adding 'a' to each row of 'b'"
]
},
{
"cell_type": "code",
"execution_count": 11,
"id": "e1bdb80f",
"metadata": {
"ExecuteTime": {
"end_time": "2023-06-27T20:08:54.384721Z",
"start_time": "2023-06-27T20:08:54.370392Z"
},
"slideshow": {
"slide_type": "fragment"
}
},
"outputs": [
{
"data": {
"text/plain": [
"array([[ 5, 7, 9],\n",
" [ 8, 10, 12]])"
]
},
"execution_count": 11,
"metadata": {},
"output_type": "execute_result"
}
],
"source": [
"a + b"
]
},
{
"cell_type": "code",
"execution_count": null,
"id": "88c2ac1b",
"metadata": {
"ExecuteTime": {
"end_time": "2023-06-27T20:08:54.837311Z",
"start_time": "2023-06-27T20:08:54.826312Z"
},
"slideshow": {
"slide_type": "fragment"
}
},
"outputs": [],
"source": [
"# I don't need to loop through each row of b and add a\n",
"print('Inefficient')\n",
"for i in b:\n",
" print(i + a)\n",
"\n",
"print()\n",
"\n",
"print('Inefficient')\n",
"#Or repeat a to match the dimensions of b\n",
"newa = np.array([[1, 2, 3], [1, 2, 3]])\n",
"newa = np.tile(a, (2, 1))\n",
"print(newa+b)"
]
},
{
"cell_type": "markdown",
"id": "f0405c0e",
"metadata": {
"slideshow": {
"slide_type": "slide"
}
},
"source": [
"The advantage of NumPy's broadcasting is that this duplication of values **does not actually take place**, but it is a useful mental model as we think about broadcasting."
]
},
{
"cell_type": "markdown",
"id": "e503116b",
"metadata": {
"slideshow": {
"slide_type": "slide"
}
},
"source": [
"## Why does understanding broadcasting matter?\n",
"+ Efficient element-wise operations with numpy\n",
"+ Simplifies code\n",
"+ Flexibly manipulate data\n",
"+ Understand broadcasting errors"
]
},
{
"cell_type": "markdown",
"id": "bd0c6d0f",
"metadata": {
"slideshow": {
"slide_type": "slide"
}
},
"source": [
"Three rules of Broadcasting\n",
"\n",
"When operating on two arrays, NumPy compares their shapes."
]
},
{
"cell_type": "markdown",
"id": "4a4810e6",
"metadata": {
"slideshow": {
"slide_type": "-"
}
},
"source": [
"Rule 1: If the two arrays differ in their number of dimensions, the shape of the one with fewer dimensions is padded with ones on its leading LEFT side : **Pad**\n",
"\n",
"Rule 2: If the shape of the two arrays does not match in any dimension, the array with shape equal to 1 in that dimension is stretched to match the other shape : **Stretch**\n",
"\n",
"Rule 3: If in any dimension the sizes disagree and neither is equal to 1, an error is raised : **Check**"
]
},
{
"cell_type": "markdown",
"id": "30bb103b",
"metadata": {
"slideshow": {
"slide_type": "fragment"
}
},
"source": [
" Pad, Stretch, Check "
]
},
{
"cell_type": "markdown",
"id": "c65b5dcf",
"metadata": {
"slideshow": {
"slide_type": "slide"
}
},
"source": [
"## Broadcasting example 1"
]
},
{
"cell_type": "code",
"execution_count": null,
"id": "b37486ef",
"metadata": {
"ExecuteTime": {
"end_time": "2023-06-27T20:16:55.153853Z",
"start_time": "2023-06-27T20:16:55.144099Z"
},
"slideshow": {
"slide_type": "fragment"
}
},
"outputs": [],
"source": [
"a = np.array([1, 2, 3])\n",
"b = np.array([[4, 5, 6], [7, 8, 9]])\n",
"\n",
"print(a)\n",
"print('')\n",
"print(b)"
]
},
{
"cell_type": "code",
"execution_count": null,
"id": "1d948492",
"metadata": {
"ExecuteTime": {
"end_time": "2023-06-27T20:16:55.456925Z",
"start_time": "2023-06-27T20:16:55.448580Z"
}
},
"outputs": [],
"source": [
"print(a.shape)\n",
"print()\n",
"print(b.shape)"
]
},
{
"cell_type": "markdown",
"id": "cd4286d9",
"metadata": {
"slideshow": {
"slide_type": "fragment"
}
},
"source": [
"### Rule 1: Pad\n",
"\n",
">If the two arrays differ in their number of dimensions, the shape of the one with fewer dimensions is padded with ones on its leading (left) side"
]
},
{
"cell_type": "markdown",
"id": "7e69836a",
"metadata": {
"slideshow": {
"slide_type": "fragment"
}
},
"source": [
"We see by rule 1 that the array a has fewer dimensions, so we pad it on the left with ones:\n",
"\n",
"a.shape -> (1, 3)\n",
"\n",
"b.shape -> (2, 3)"
]
},
{
"cell_type": "markdown",
"id": "e5219f11",
"metadata": {
"slideshow": {
"slide_type": "fragment"
}
},
"source": [
"### Rule 2: Stretch\n",
"\n",
"> If the shape of the two arrays does not match in any dimension, the array with shape equal to 1 in that dimension is stretched or \"broadcast\" to match the other shape.\n"
]
},
{
"cell_type": "markdown",
"id": "f434a4d9",
"metadata": {
"slideshow": {
"slide_type": "fragment"
}
},
"source": [
"By rule 2, we now see that the first dimension disagrees, so we stretch this dimension in a to match:\n",
"\n",
"a.shape -> (2, 3)\n",
"\n",
"b.shape -> (2, 3)\n",
"\n",
"The shapes match, and we see that the final shape will be (2, 3)"
]
},
{
"cell_type": "markdown",
"id": "2fac50f0",
"metadata": {
"slideshow": {
"slide_type": "fragment"
}
},
"source": [
"### Rule 3: Check\n",
"\n",
"> If in any dimension the sizes disagree and neither is equal to 1, an error is raised : Check"
]
},
{
"cell_type": "code",
"execution_count": null,
"id": "3545ef93",
"metadata": {
"ExecuteTime": {
"end_time": "2023-06-27T20:13:25.685732Z",
"start_time": "2023-06-27T20:13:25.675680Z"
},
"slideshow": {
"slide_type": "fragment"
}
},
"outputs": [],
"source": [
"(a+b).shape"
]
},
{
"cell_type": "markdown",
"id": "a718ef31",
"metadata": {
"slideshow": {
"slide_type": "slide"
}
},
"source": [
"## Broadcasting example 2"
]
},
{
"cell_type": "code",
"execution_count": null,
"id": "be17841c",
"metadata": {
"ExecuteTime": {
"end_time": "2023-06-27T20:29:24.820033Z",
"start_time": "2023-06-27T20:29:24.813695Z"
}
},
"outputs": [],
"source": [
"a = np.arange(3)\n",
"b = np.arange(4).reshape(4, 1)"
]
},
{
"cell_type": "code",
"execution_count": null,
"id": "419709a9",
"metadata": {
"ExecuteTime": {
"end_time": "2023-06-27T20:29:25.101341Z",
"start_time": "2023-06-27T20:29:25.094246Z"
}
},
"outputs": [],
"source": [
"print(a.shape)\n",
"print()\n",
"print(b.shape)"
]
},
{
"cell_type": "code",
"execution_count": null,
"id": "a583f31c",
"metadata": {
"ExecuteTime": {
"end_time": "2023-06-27T20:29:25.391210Z",
"start_time": "2023-06-27T20:29:25.381999Z"
}
},
"outputs": [],
"source": [
"(a+b).shape"
]
},
{
"cell_type": "markdown",
"id": "53603d10",
"metadata": {
"slideshow": {
"slide_type": "slide"
}
},
"source": [
"## Broadcasting example 3"
]
},
{
"cell_type": "code",
"execution_count": 12,
"id": "1001798b",
"metadata": {
"ExecuteTime": {
"end_time": "2023-06-27T20:29:26.282334Z",
"start_time": "2023-06-27T20:29:26.276961Z"
},
"slideshow": {
"slide_type": "fragment"
}
},
"outputs": [],
"source": [
"a = np.ones((3, 2))\n",
"b = np.array([4, 5, 6])"
]
},
{
"cell_type": "code",
"execution_count": 13,
"id": "6969597a",
"metadata": {
"ExecuteTime": {
"end_time": "2023-06-27T20:29:26.524280Z",
"start_time": "2023-06-27T20:29:26.514221Z"
},
"slideshow": {
"slide_type": "-"
}
},
"outputs": [
{
"name": "stdout",
"output_type": "stream",
"text": [
"(3, 2)\n",
"\n",
"(3,)\n"
]
}
],
"source": [
"print(a.shape)\n",
"print()\n",
"print(b.shape)"
]
},
{
"cell_type": "code",
"execution_count": 14,
"id": "adabdf3d",
"metadata": {
"ExecuteTime": {
"end_time": "2023-06-27T20:29:27.021351Z",
"start_time": "2023-06-27T20:29:27.006776Z"
},
"slideshow": {
"slide_type": "-"
},
"tags": [
"raises-exception"
]
},
"outputs": [
{
"ename": "ValueError",
"evalue": "operands could not be broadcast together with shapes (3,2) (3,) ",
"output_type": "error",
"traceback": [
"\u001b[0;31m---------------------------------------------------------------------------\u001b[0m",
"\u001b[0;31mValueError\u001b[0m Traceback (most recent call last)",
"Cell \u001b[0;32mIn[14], line 1\u001b[0m\n\u001b[0;32m----> 1\u001b[0m (\u001b[43ma\u001b[49m\u001b[38;5;241;43m+\u001b[39;49m\u001b[43mb\u001b[49m)\u001b[38;5;241m.\u001b[39mshape\n",
"\u001b[0;31mValueError\u001b[0m: operands could not be broadcast together with shapes (3,2) (3,) "
]
}
],
"source": [
"(a+b).shape"
]
},
{
"cell_type": "markdown",
"id": "c48fa3cb",
"metadata": {
"slideshow": {
"slide_type": "fragment"
}
},
"source": [
"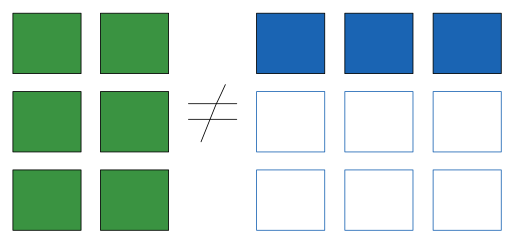"
]
},
{
"cell_type": "markdown",
"id": "9aa28998",
"metadata": {
"slideshow": {
"slide_type": "fragment"
}
},
"source": [
"### Rule 3 : Check\n",
">If in any dimension the sizes disagree and neither is equal to 1, an error is raised."
]
},
{
"cell_type": "markdown",
"id": "2d84ff92",
"metadata": {
"slideshow": {
"slide_type": "fragment"
}
},
"source": [
"But numpy should have just padded on the right....\n",
">thats not how the broadcasting rules work! It would lead to potential areas of ambiguity. If right-side padding is what you'd like, you can do this explicitly by reshaping the array "
]
},
{
"cell_type": "code",
"execution_count": 15,
"id": "103cdcdb",
"metadata": {
"ExecuteTime": {
"end_time": "2023-06-27T20:34:53.502018Z",
"start_time": "2023-06-27T20:34:53.496501Z"
},
"slideshow": {
"slide_type": "slide"
}
},
"outputs": [],
"source": [
"a = np.ones((3, 2))\n",
"b = np.array([4, 5, 6])[:, np.newaxis]\n",
"# b = np.array([4, 5, 6]).reshape((3, 1))"
]
},
{
"cell_type": "code",
"execution_count": 16,
"id": "847e919f",
"metadata": {
"ExecuteTime": {
"end_time": "2023-06-27T20:34:53.940537Z",
"start_time": "2023-06-27T20:34:53.932615Z"
},
"slideshow": {
"slide_type": "fragment"
}
},
"outputs": [
{
"name": "stdout",
"output_type": "stream",
"text": [
"(3, 2)\n",
"\n",
"(3, 1)\n"
]
}
],
"source": [
"print(a.shape)\n",
"print()\n",
"print(b.shape)"
]
},
{
"cell_type": "code",
"execution_count": 17,
"id": "fb7cd974",
"metadata": {
"ExecuteTime": {
"end_time": "2023-06-27T20:34:54.863120Z",
"start_time": "2023-06-27T20:34:54.849693Z"
},
"slideshow": {
"slide_type": "fragment"
}
},
"outputs": [
{
"data": {
"text/plain": [
"(3, 2)"
]
},
"execution_count": 17,
"metadata": {},
"output_type": "execute_result"
}
],
"source": [
"(a+b).shape"
]
},
{
"cell_type": "markdown",
"id": "7f3dffb8",
"metadata": {
"slideshow": {
"slide_type": "fragment"
}
},
"source": [
"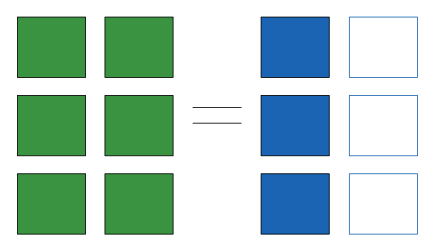"
]
},
{
"cell_type": "markdown",
"id": "c0c21eac",
"metadata": {
"slideshow": {
"slide_type": "slide"
}
},
"source": [
"### Recap"
]
},
{
"cell_type": "markdown",
"id": "cf9e9234",
"metadata": {
"slideshow": {
"slide_type": "-"
}
},
"source": [
"```\n",
"Scalar 2D 3D Bad\n",
"\n",
"( ,) (3, 4) (3, 5, 1) (3, 5, 2)\n",
"(3,) (3, 1) ( 8) ( 8)\n",
"---- ------ --------- ---------\n",
"(3,) (3, 4) (3, 5, 8) XXX\n",
"```"
]
},
{
"cell_type": "markdown",
"id": "6cd0f8cf",
"metadata": {
"slideshow": {
"slide_type": "slide"
}
},
"source": [
" Mind-on exercises "
]
},
{
"cell_type": "markdown",
"id": "acba732f",
"metadata": {
"slideshow": {
"slide_type": "slide"
}
},
"source": [
"### Exercise 1: warm up\n",
"\n",
"```What is the expected output shape for each operation?```"
]
},
{
"cell_type": "code",
"execution_count": 19,
"id": "a41d0f74",
"metadata": {
"ExecuteTime": {
"end_time": "2023-06-27T19:58:58.881059Z",
"start_time": "2023-06-27T19:58:57.830Z"
},
"slideshow": {
"slide_type": "fragment"
}
},
"outputs": [
{
"data": {
"text/plain": [
"(5,)"
]
},
"execution_count": 19,
"metadata": {},
"output_type": "execute_result"
}
],
"source": [
"a = np.arange(5)\n",
"b = 5\n",
"\n",
"np.shape(a-b)"
]
},
{
"cell_type": "code",
"execution_count": 21,
"id": "6f82a2fb",
"metadata": {
"ExecuteTime": {
"end_time": "2023-06-27T19:58:58.884966Z",
"start_time": "2023-06-27T19:58:57.833Z"
},
"slideshow": {
"slide_type": "fragment"
}
},
"outputs": [
{
"data": {
"text/plain": [
"(7, 7)"
]
},
"execution_count": 21,
"metadata": {},
"output_type": "execute_result"
}
],
"source": [
"a = np.ones((7, 1))\n",
"b = np.arange(7)\n",
"np.shape(a*b)"
]
},
{
"cell_type": "code",
"execution_count": 23,
"id": "808095ad",
"metadata": {
"ExecuteTime": {
"end_time": "2023-06-27T19:58:58.888119Z",
"start_time": "2023-06-27T19:58:57.836Z"
},
"slideshow": {
"slide_type": "fragment"
}
},
"outputs": [
{
"data": {
"text/plain": [
"(2, 3, 3)"
]
},
"execution_count": 23,
"metadata": {},
"output_type": "execute_result"
}
],
"source": [
"a = np.random.randint(0, 50, (2, 3, 3))\n",
"b = np.random.randint(0, 10, (3, 1))\n",
"\n",
"np.shape(a-b)"
]
},
{
"cell_type": "code",
"execution_count": 25,
"id": "d9a12a90",
"metadata": {
"ExecuteTime": {
"end_time": "2023-06-27T19:58:58.891462Z",
"start_time": "2023-06-27T19:58:57.839Z"
},
"slideshow": {
"slide_type": "fragment"
}
},
"outputs": [
{
"ename": "ValueError",
"evalue": "operands could not be broadcast together with shapes (10,10) (9,) ",
"output_type": "error",
"traceback": [
"\u001b[0;31m---------------------------------------------------------------------------\u001b[0m",
"\u001b[0;31mValueError\u001b[0m Traceback (most recent call last)",
"Cell \u001b[0;32mIn[25], line 4\u001b[0m\n\u001b[1;32m 1\u001b[0m a \u001b[38;5;241m=\u001b[39m np\u001b[38;5;241m.\u001b[39marange(\u001b[38;5;241m100\u001b[39m)\u001b[38;5;241m.\u001b[39mreshape(\u001b[38;5;241m10\u001b[39m, \u001b[38;5;241m10\u001b[39m)\n\u001b[1;32m 2\u001b[0m b \u001b[38;5;241m=\u001b[39m np\u001b[38;5;241m.\u001b[39marange(\u001b[38;5;241m1\u001b[39m, \u001b[38;5;241m10\u001b[39m)\n\u001b[0;32m----> 4\u001b[0m np\u001b[38;5;241m.\u001b[39mshape(\u001b[43ma\u001b[49m\u001b[38;5;241;43m+\u001b[39;49m\u001b[43mb\u001b[49m)\n",
"\u001b[0;31mValueError\u001b[0m: operands could not be broadcast together with shapes (10,10) (9,) "
]
}
],
"source": [
"a = np.arange(100).reshape(10, 10)\n",
"b = np.arange(1, 10)\n",
"\n",
"np.shape(a+b)"
]
},
{
"cell_type": "markdown",
"id": "69632f95",
"metadata": {
"slideshow": {
"slide_type": "slide"
}
},
"source": [
"### Exercise 2\n",
"\n",
"```\n",
"1. Create a random 2D array of dimension (5, 3)\n",
"2. Calculate the maximum value of each row\n",
"3. Divide each row by its maximum\n",
"```\n",
"\n",
"Remember to use broadcasting : NO FOR LOOPS!"
]
},
{
"cell_type": "code",
"execution_count": null,
"id": "54e2a53e",
"metadata": {
"ExecuteTime": {
"end_time": "2023-06-27T19:58:58.894433Z",
"start_time": "2023-06-27T19:58:57.843Z"
},
"slideshow": {
"slide_type": "fragment"
}
},
"outputs": [],
"source": [
"## Your code here"
]
},
{
"cell_type": "markdown",
"id": "b9facc0f",
"metadata": {
"slideshow": {
"slide_type": "slide"
}
},
"source": [
"### Exercise 3"
]
},
{
"cell_type": "markdown",
"id": "7e8156d0",
"metadata": {
"slideshow": {
"slide_type": "fragment"
}
},
"source": [
"Task: Find the closest **cluster** to the **observation**. \n",
"\n",
"Again, use broadcasting: DO NOT iterate cluster by cluster"
]
},
{
"cell_type": "code",
"execution_count": null,
"id": "2969994e",
"metadata": {
"ExecuteTime": {
"end_time": "2023-06-27T19:58:58.899204Z",
"start_time": "2023-06-27T19:58:57.847Z"
},
"slideshow": {
"slide_type": "fragment"
}
},
"outputs": [],
"source": [
"observation = np.array([30.0, 99.0]) #Observation\n",
"\n",
"#Clusters\n",
"clusters = np.array([[102.0, 203.0],\n",
" [132.0, 193.0],\n",
" [45.0, 155.0], \n",
" [57.0, 173.0]])"
]
},
{
"cell_type": "markdown",
"id": "f13352ff",
"metadata": {
"slideshow": {
"slide_type": "fragment"
}
},
"source": [
"Lets plot this data\n",
"\n",
"In the plot below, **+** is the observation and dots are the cluster coordinates"
]
},
{
"cell_type": "code",
"execution_count": null,
"id": "b9f6b5cf",
"metadata": {
"ExecuteTime": {
"end_time": "2023-06-27T19:58:58.906715Z",
"start_time": "2023-06-27T19:58:57.850Z"
},
"slideshow": {
"slide_type": "fragment"
}
},
"outputs": [],
"source": [
"import matplotlib.pyplot as plt \n",
"\n",
"plt.scatter(clusters[:, 0], clusters[:, 1]) #Scatter plot of clusters\n",
"for n, x in enumerate(clusters):\n",
" print('cluster %d' %n)\n",
" plt.annotate('cluster%d' %n, (x[0], x[1])) #Label each cluster\n",
"plt.plot(observation[0], observation[1], '+'); #Plot observation"
]
},
{
"cell_type": "markdown",
"id": "4f9b84e2",
"metadata": {
"slideshow": {
"slide_type": "fragment"
}
},
"source": [
"Closest cluster as seen by the plot is **2**. Your task is to write a function to calculate this"
]
},
{
"cell_type": "markdown",
"id": "8aea6781",
"metadata": {
"ExecuteTime": {
"end_time": "2023-06-26T19:25:08.202848Z",
"start_time": "2023-06-26T19:25:08.194923Z"
}
},
"source": [
"\n",
"**hint:** Find the distance between the observation and each row in the cluster. The cluster to which the observation belongs to is the row with the minimum distance.\n",
"\n",
"distance = $\\sqrt {\\left( {x_1 - x_2 } \\right)^2 + \\left( {y_1 - y_2 } \\right)^2 }$"
]
},
{
"cell_type": "code",
"execution_count": null,
"id": "ea8a7240",
"metadata": {
"ExecuteTime": {
"end_time": "2023-06-27T19:58:58.916610Z",
"start_time": "2023-06-27T19:58:57.854Z"
},
"slideshow": {
"slide_type": "fragment"
}
},
"outputs": [],
"source": [
"## Your code here"
]
},
{
"cell_type": "markdown",
"id": "beaee243",
"metadata": {
"slideshow": {
"slide_type": "skip"
}
},
"source": [
"## Sources + Resources\n",
"\n",
"ASPP 2016 - Stéfan van der Walt - https://github.com/ASPP/2016_numpy\n",
"\n",
"Basic Numpy: http://scipy-lectures.org/intro/numpy/index.html\n",
"\n",
"Advanced Numpy: http://scipy-lectures.org/advanced/advanced_numpy/index.html\n",
"\n",
"Numpy chapter in \"Python Data Science Handbook\" https://jakevdp.github.io/PythonDataScienceHandbook/02.00-introduction-to-numpy.html"
]
}
],
"metadata": {
"celltoolbar": "Slideshow",
"kernelspec": {
"display_name": "Python 3 (ipykernel)",
"language": "python",
"name": "python3"
},
"language_info": {
"codemirror_mode": {
"name": "ipython",
"version": 3
},
"file_extension": ".py",
"mimetype": "text/x-python",
"name": "python",
"nbconvert_exporter": "python",
"pygments_lexer": "ipython3",
"version": "3.11.3"
},
"rise": {
"scroll": true
},
"toc": {
"base_numbering": 1,
"nav_menu": {},
"number_sections": true,
"sideBar": true,
"skip_h1_title": false,
"title_cell": "Table of Contents",
"title_sidebar": "Contents",
"toc_cell": false,
"toc_position": {},
"toc_section_display": true,
"toc_window_display": false
}
},
"nbformat": 4,
"nbformat_minor": 5
}